【交流】Nature Reviews Neuroscience 前任编辑 Dr. McGowan论文写作系列第九讲:让统计学告诉你的结果意味着什么?
Statistics: what can we say about our findings?

Dr. Daniel McGowan
分子神经科学博士
Dr. Daniel McGowan曾任 Nature Reviews Neuroscience 副编辑,负责约稿,管理和撰写期刊内容。于2006年加入理文编辑(Edanz Group) 并从2008年起担任学术总监。Dr. McGowan有超过十年的博士后和研究生阶段实验室研究经验,主要致力于神经退化疾病、分子及细胞生物学、蛋白质生物化学、蛋白质组学和基因组学。
精彩回顾
第一期:Dr. McGowan论文写作系列第一讲: “How to write a world class paper”(标题练习)
第二期: Dr. McGowan论文写作系列第二讲:“摘要和关键词(附PPT和在线修改)”
第三期: Dr. McGowan论文写作系列第三讲:“cover letter的练习”
第四期: Dr. McGowan论文写作系列第四讲:“Nature Reviews Neuroscience 前任编辑帮您修改语言问题”
第五期: Dr. McGowan论文写作系列第五讲:“如何做好研究设计和预先规划工作。 ”
第六期: Dr. McGowan论文写作系列第六讲:“如何选择合适的目标期刊?”
第七期: Dr. McGowan论文写作系列第七讲:“如何撰写规范的IMRaD格式?”
第八期: Dr. McGowan论文写作系列第八讲:“如何完成一个出色的论文图表?”
第九期: Dr. McGowan论文写作系列第九讲:“让统计学告诉你的结果意味着什么?”
第十期: Dr. McGowan论文写作系列第十讲:“如何回复审稿意见? ”
第十一期: Dr. McGowan论文写作系列第十一讲:“科研伦理和论文发表道德”
第十二期: Dr. McGowan论文写作系列第十二讲:“投稿,最后检查”
编者按:在本帖中,理文编辑学术总监Dr. Daniel McGowan将向大家展示:“统计学:告诉你的结果意味着什么?”
统计学:告诉你的结果意味着什么?
Dr. McGowan论文写作系列第九讲 — Statistics: what can we say about our findings?
Today, few professional activities are untouched by statistical thinking, and most academic disciplines use it to a greater or lesser degree… Statistics has developed out of an aspect of our everyday thinking to be a ubiquitous tool of systematic research… Statistical thinking is a way of recognizing that our observations of the world can never be totally accurate; they are always somewhat uncertain.
Rowntree D (1981). Statistics without tears. A primer for non-mathematicians. Penguin Books Ltd., London, England.
The term ‘statistics’ refers to the methods used to collect, process and interpret data. Because these methods are so inherent in the process of scientific inquiry, there have been multiple references to statistics throughout this tips series, namely, in the tips on study design, methods, results and display items. However, given the importance of statistics in most scientific studies, it is worthwhile having a separate tip on how they should be used and presented.
统计学是如此重要,贯穿了科学研究中的设计、方法、结果和展示等全过程,因此很必要把统计的问题单独拎出来讲讲。
Statistics should first be considered long before the commencement of any research, during the initial study design. First, consider what information you need to collect in order to test your hypothesis or address your research question.(开始实验前,请先考虑实验中的那些数据将被统计?) It is important to get this right from the outset because, while data can be reanalyzed relatively easily if the wrong tests were used, it is far more difficult and time-consuming to repeat data collection with a different sample group or obtain additional variables from the same sample. If you wish to test the efficacy of a treatment for use in the general population, then your sample needs to be representative of the general population.(选择有代表性的样品) If you wish to test its efficacy in a given ethnicity or age group, then your sample needs to be representative of that group. If comparing two groups of subjects separated on the basis of a particular disease or behavior, then other variables, such as age, sex and ethnicity, need to be matched as closely as possible between the two groups.(控制主要变量的一致性) This aspect of statistics relates to the collection of data; get it wrong and you could face major problems, potentially the need to start the research all over again, at the peer review stage many months later.
Second, you need to consider what statistical tests should be applied so that you can make meaningful statements about your data.(选择合适的统计方法来解释数据。) This depends on the type of data you have collected(区别数据类型:等级资料还是计量资料。): do you have categorical data, perhaps describing the presence or absence of a particular marker, or quantitative data with numerical values? If your data is quantitative, is it continuous (that is, can it be measured) or discrete (counts)? For example, age, weight, time and temperature are all examples of continuous data because they are measured on continuous scales with units that are infinitely sub-divisible. By contrast, the number of people in a given group and the number of cells with apoptotic features are examples of discrete data that need to be counted and are not sub-divisible. You also need to know how your data is distributed: is it normally distributed (Gaussian) or skewed? (区别数据分布类型:正态分布还是非正态分布)This also affects the type of test that should be used. It is important that you know what type of data you are collecting so that you apply the appropriate statistical tests to analyze the data and so you present them in an appropriate manner.
下面介绍一个很实用的统计学习网站
The following useful website provides a guide to choosing the appropriate statistical test:
http://www.graphpad.com/www/Book/Choose.htm
Finally, you need to know how to interpret the results of the statistical tests you have selected. (解释统计数据)What exactly does the p (or t or χ2 or other) value mean? That, after all is the point of statistical analysis: to determine what you can say about your findings, what they really mean. (统计分析的真正意义!)Statistics enable us to determine the central tendency (for example, mean and median) and dispersion (for example, standard deviation, standard error, and interpercentile range) of a dataset, giving us an idea of its distribution. Also using statistics, values from two or more different sample groups can be compared (for example, by t-test, analysis of variance, or χ2 test) to determine if a difference between or among groups could have arisen by chance. If this hypothesis, known as the null hypothesis, can be shown to be unlikely, then the difference is said to be significant. It is important to keep in mind that there are two risks associated with reducing a decision about the ‘reality’ of a difference to probabilities, and both depend on the threshold set to determine significance: the first, known as type I error, is the possibility that a difference is accepted as significant when it is not; the opposite risk, known as type II error, refers to the possibility that a significant difference is considered not to be significant because we demand a larger difference between groups to be certain. Reducing the risk of type I errors increases the risk of type II errors, but this is infinitely more preferable than reaching a conclusion that isn’t justified. Statistics also provides a measure of the strengths of correlations and enables inferences about a much larger population to be drawn on the basis of findings in a sample group. In this way, statistics puts meaning into findings that would otherwise be of limited value, and allows us to draw conclusions based on probabilities, even when the possibility of error remains.
现在,大家来看以下的例子:
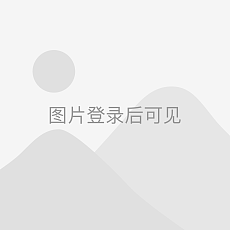
Extracts from The Journal of Clinical Investigation (doi:10.1172/JCI38289; reproduced with permission).
Checklist 备忘录
1. Indicate what parameters are described when listing data; for example, “means±S.D.”
2. Indicate the statistical tests used to analyze data
3. Give the numerator and denominator with percentages; for example “40% (100/250)”
4. Use means and standard deviations to report normally distributed data
5. Use medians and interpercentile ranges to report data with a skewed distribution
6. Report p values; for example, use “p=0.0035” rather than “p<0.05”
7. Only use the word “significant’ when describing statistically significant differences.
请大家注意,尽管理文编辑(Edanz Group)设在中国,Dr. McGowan的母语是英语,因此请各位尽量使用英语交流,这也是一个很好的锻炼机会!
更多精彩内容请下载完整的“Writing for Success”系列培训材料。
最后编辑于 2010-04-06 · 浏览 5811